This is the first installment of a six-part series exploring how AI is transforming medical research and healthcare.
Before me is a beating, computer-generated heart—an artificial “digital twin.” While it mimics the movements of a human heart, it lacks blood and isn’t housed within a body. This digital twin is used to test cardiovascular devices like stents and prosthetic valves, ensuring their safety before application in real patients.
The creators, Adsilico, have advanced beyond a single model. Harnessing artificial intelligence and vast datasets, they’ve developed numerous virtual hearts. These AI-driven hearts represent various biological traits such as age, weight, gender, blood pressure, health conditions, and ethnic backgrounds. This diversity addresses gaps often overlooked in clinical trials and traditional digital twin testing.
“This approach captures the full range of patient anatomies and physiological responses, which is unachievable through conventional methods. By incorporating AI, we enhance testing, leading to safer and more inclusive devices,” says Adsilico CEO Sheena Macpherson. She highlights how, in 2018, a report by the International Consortium of Investigative Journalists revealed over 83,000 deaths and 1.7 million injuries linked to medical devices.
Macpherson envisions AI-powered digital twins reducing these statistics significantly. “Thorough testing is key to improving safety, but clinical trials are often prohibitively expensive. With virtual models, we can rigorously test devices before they are used in humans,” she explains.
By using digital twins, manufacturers can simulate diverse conditions like varying blood pressure levels or disease progressions, gaining critical insights. “This level of detail is invaluable,” says Macpherson. “It also enables testing on previously underrepresented groups, moving beyond the traditional focus on white men in clinical studies.”
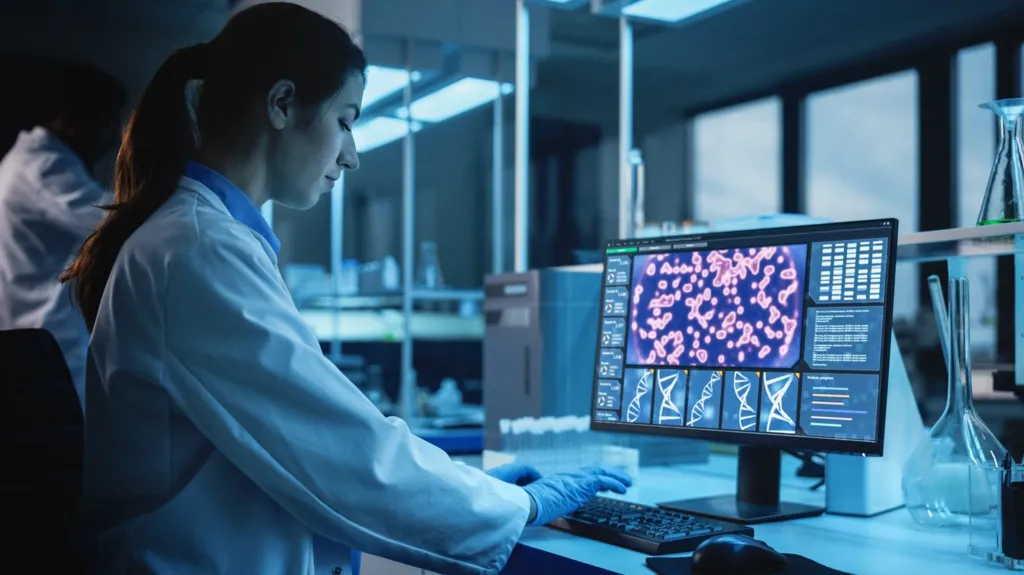
AI can spot patterns that humans might miss
Adsilico trains its AI models using a combination of cardiovascular data and MRI/CT scans obtained with patient consent. These datasets provide detailed anatomical insights, enabling the creation of precise digital representations of how medical devices interact with varying heart anatomies.
The testing process involves generating a digital twin of the medical device, which is then virtually implanted into an AI-simulated heart. Unlike traditional human or animal trials, which typically involve hundreds of participants, this digital approach allows the testing to scale across thousands of virtual hearts—replicating diverse patient anatomies and conditions entirely within a computer.
A key advantage for drug and device manufacturers is the significant reduction in time and cost. For instance, pharmaceutical company Sanofi aims to cut testing durations by 20% while improving success rates by integrating digital twin technology into its immunology, oncology, and rare disease research.
Sanofi’s approach uses biological data to create AI-based simulated patients, not clones of real individuals, which are distributed across trial control and placebo groups. Additionally, AI generates virtual models of the drugs being tested, simulating properties such as absorption and predicting patient reactions. This replicates real-world trial scenarios, enabling more efficient and comprehensive testing processes.
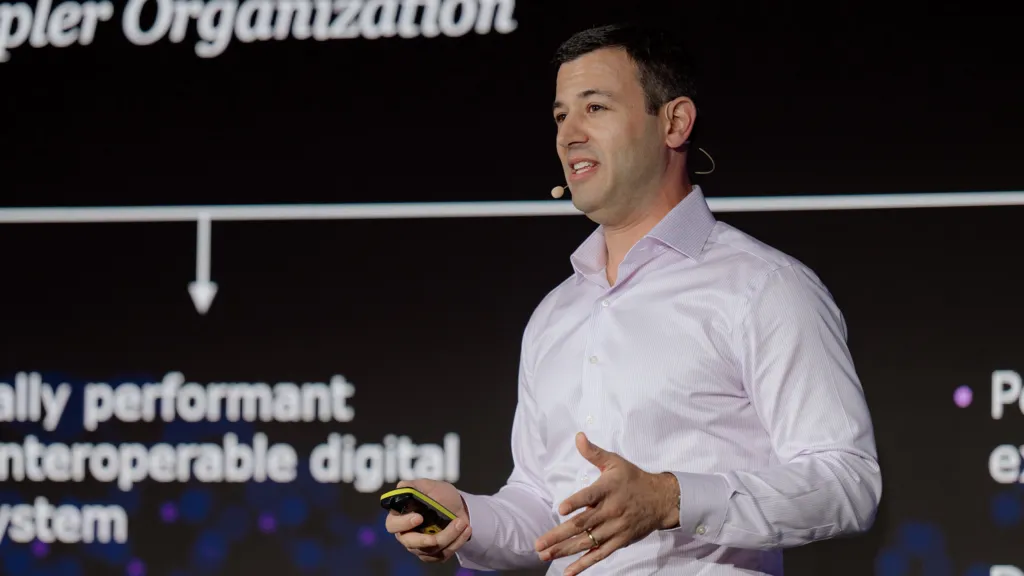
Using digital twins could mean big savings for drugs firms says Matt Truppo
Matt Truppo, Sanofi’s global head of research platforms and computational R&D, emphasizes that digital twins have the potential to drive significant savings in drug development. “With a 90% failure rate for new drugs during clinical trials, even a 10% increase in success rates through technologies like digital twins could save $100 million, given the high costs of late-phase trials,” he explains.
Based in Boston, Truppo notes that early outcomes have been encouraging but acknowledges the challenges ahead. “There’s still much to be done. Many of the diseases we aim to tackle are extremely complex. This is where AI becomes essential,” he says. Truppo sees the integration of advanced AI models with digital twins as the next major step in addressing intricate human biological processes and transforming drug discovery.
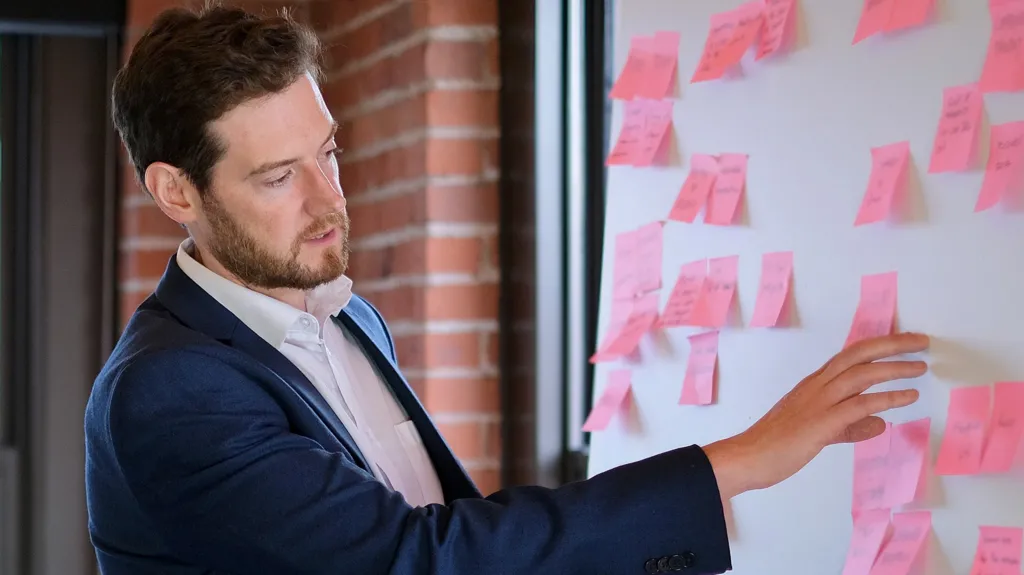
AI is only as good as the data sets it is trained on says Charlie Paterson
Charlie Paterson, an associate partner at PA Consulting and former NHS service manager, highlights potential weaknesses of digital twins, noting that their effectiveness depends on the quality of the data they are trained with. He warns that outdated data collection methods and underrepresentation of marginalized groups could introduce biases when creating virtual representations of individuals.
Sanofi acknowledges the challenge of working with limited legacy data to train its AI and is actively addressing it. To supplement its internal data, which includes millions of data points from patients in clinical trials, the company uses third-party sources like electronic health records and biobanks.
At Adsilico, Ms. Macpherson remains optimistic that AI-driven digital twin technology will eventually replace animal testing in clinical trials, which is still deemed essential in drug and device testing. She points out that virtual heart models are closer to human hearts than those of animals typically used in implantable device studies, such as dogs, cows, sheep, or pigs.