Researchers suggest that Artificial Intelligence has the potential to save lives by providing advanced warnings about the precise locations where hurricanes will make landfall, surpassing the capabilities of traditional forecasting systems.
A recent AI tool developed by Google DeepMind successfully forecasted the location of Hurricane Lee’s landfall in Canada three days earlier than conventional methods. Over the years, weather predictions have significantly improved in accuracy. However, scientists believe that AI’s rapid processing and its capacity to analyze historical data for predictions mark a significant advancement, making it a transformative force in weather forecasting.
A precise weather prediction serves the purpose of guiding daily attire choices and, more significantly, serves as an early warning system for severe weather events like storms, floods, and heatwaves. This forewarning allows communities crucial time to prepare.
Conventional weather forecasts demand extensive computing resources. They entail estimating numerous variables such as air pressure, temperature, wind speeds, and humidity at various atmospheric levels worldwide.
Google DeepMind introduced a new AI tool called GraphCast, which surpasses the European Medium Range Weather Forecasting model, considered one of the world’s finest, in over 90% of these factors. This achievement was reported in a peer-reviewed paper by DeepMind in the journal Science.
GraphCast delivers its forecasts in less than a minute, utilizing significantly less computing power compared to traditional methods. This efficiency stems from its distinctive approach to forecasting.
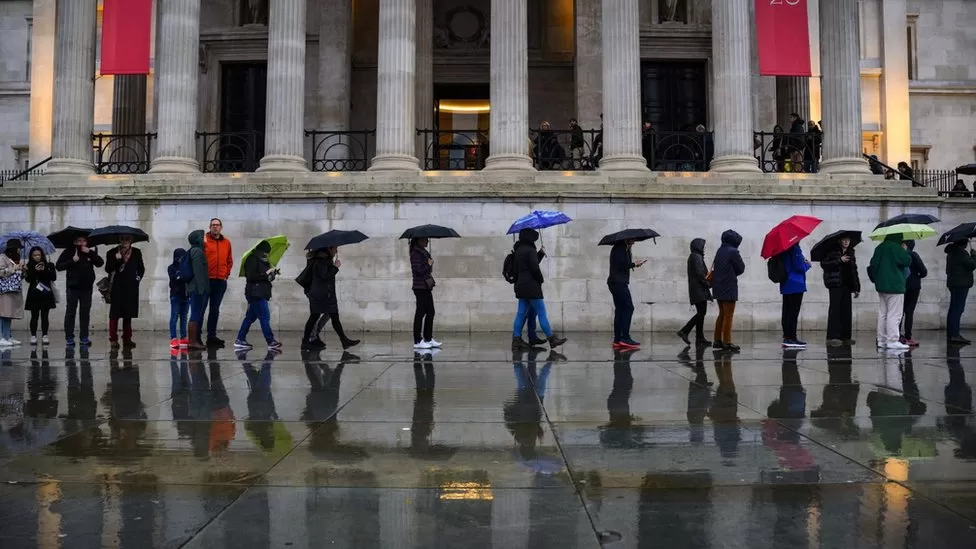
Weather forecasts not only help us plan for a rainy day but also serve as critical warnings for potentially life-threatening weather occurrences.
Traditional weather forecasting involves collecting real-time atmospheric data from various sources such as weather stations, satellites, atmospheric balloons, ocean buoys, and sensors on commercial airplanes. This data, amounting to hundreds of millions of readings globally every day, undergoes a selection process to determine its significance for forecasting.
Matthew Chantry from the European Centre for Medium Range Weather Forecasting (ECMWF) explains that approximately 10 million selected measurements contribute to one forecast generated by their model. Subsequently, this vast dataset is processed by supercomputers capable of executing quadrillions of calculations per second. These computations utilize intricate equations to simulate atmospheric changes, predicting how the weather will evolve over time.
This method has proven highly successful, significantly enhancing forecast accuracy as models improved and computing power advanced over the years. However, these numerical weather prediction (NWP) models demand immense computational resources, relying on some of the world’s largest supercomputers and typically requiring hours to generate forecasts.
An Innovative Method Has Emerged.
AI streamlines a significant portion of this process by avoiding the attempt to replicate the intricate workings of the world.
GraphCast, instead, employs machine learning techniques to process extensive historical data, including the ECMWF model’s outputs, to comprehend the evolution of weather patterns. Leveraging this knowledge, it forecasts the likely changes in present weather conditions.
This approach has demonstrated remarkable effectiveness. Remy Lam from Google DeepMind, involved in creating the weather tool, emphasizes its exceptional accuracy, derived from learning patterns across decades of data. He highlights its superiority over the industry’s standard, owing to its reliance on historical learning rather than complex equation-solving.
By sidestepping the need to solve complex equations, GraphCast generates forecasts swiftly and with significantly reduced computational requirements. While GraphCast’s forecasts might lack the detail of those produced by the ECMWF, it excels in predicting severe events such as extreme temperatures and accurately tracking the paths of major storms.
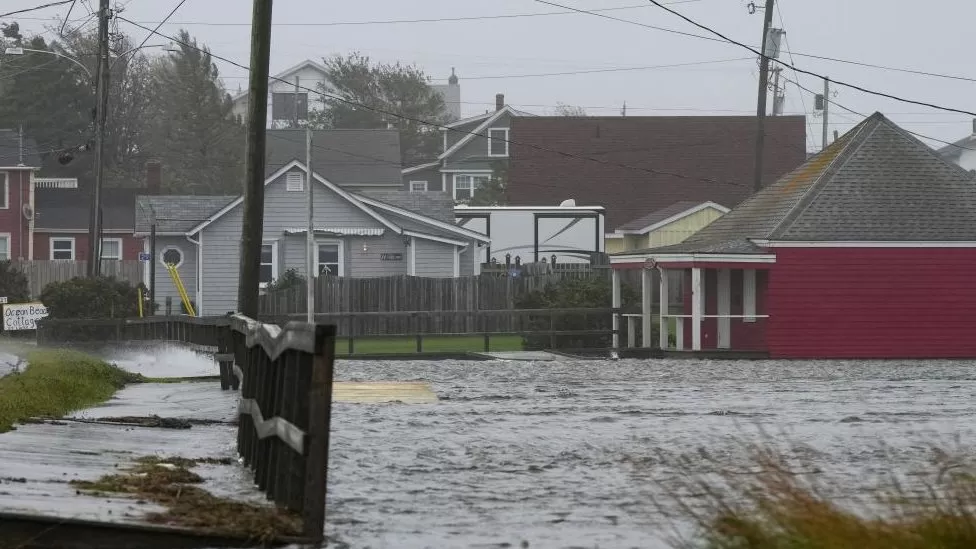
The prediction of Hurricane Lee’s landfall occurring earlier than expected.
DeepMind’s AI tool accurately forecasted the trajectory of Hurricane Lee, a storm that struck the Atlantic coast of the US and Canada in September. It notably predicted the storm’s path nine days in advance, surpassing the ECMWF’s capability, which managed only a six-day prediction window.
However, despite GraphCast’s success, it doesn’t imply that we can abandon supercomputers and solely depend on AI. Even Remy Lam from Google DeepMind acknowledges that such a shift is unlikely to occur.
“Significant Advancements”
“He says that we’re building these models on the foundation laid by previous work. Rather than supplanting traditional weather forecasts, AI models will complement them,” he believes.
“AI models rely on data, and that data originates from conventional approaches. Hence, the traditional approach remains necessary to gather data for training the model,” Mr. Lam explains.
Google DeepMind has made GraphCast open-source, allowing anyone to access the design details and utilize the technology.
Numerous technology firms and weather/climate organizations globally are developing their AI-based weather prediction tools. For instance, the UK’s national weather service, the Met Office, collaborates with the Turing Institute, the nation’s data science center, to explore AI’s potential in enhancing weather forecasting.
Acknowledging the strides made in weather forecasting with artificial intelligence and machine learning, Prof. Simon Vosper, the Met Office’s Director of Science, notes, “Weather predictions driven by AI are making significant progress.”
However, he cautions that the predictive abilities of AI-based tools might be constrained by climate change. “We’re witnessing new climate-induced weather extremes, such as the UK’s 40°C temperatures last year, which were previously unprecedented,” Prof. Vosper states.
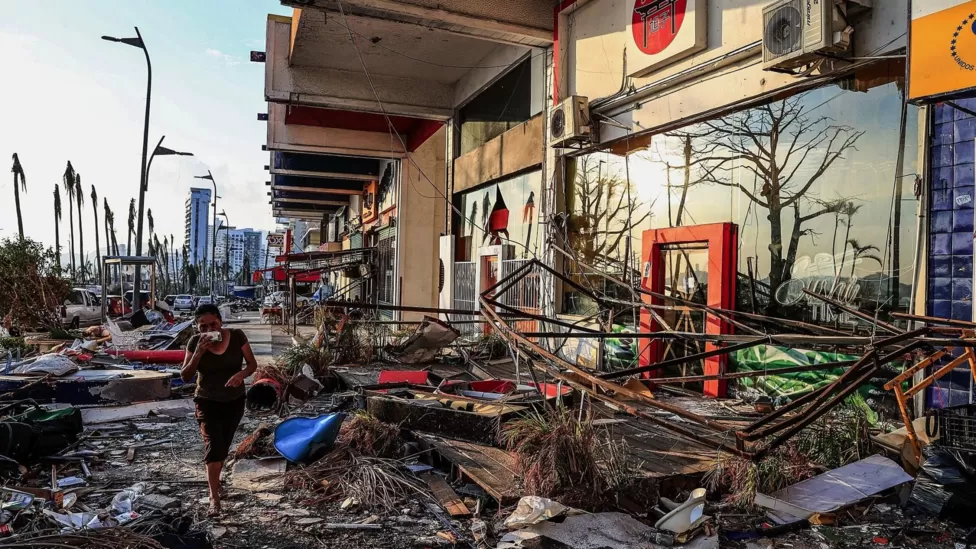
Hurricane Otis rapidly intensified from a lower category to a category five storm within a mere 24-hour period.
The manner in which severe weather systems develop might be undergoing changes.
Hurricane Otis remarkably escalated from a tropical storm to the most robust category 5 hurricane within a mere 24-hour period in October, causing severe damage upon reaching the southern coast of Mexico.
Climate scientists caution that the increasing ocean temperatures are likely to contribute to a more frequent occurrence of this rapid intensification process in storms.
Professor Vosper raises a pertinent concern: whether AI-based systems can effectively identify and forecast new extremes, given that these systems have primarily been trained on past weather conditions.